Analyzing partitioned FAIR health data responsibly
The scientific challenge of this project is to combine and learn from access-restricted FAIR health and socioeconomic data across entities in a privacy-preserving manner.
Abstract
Since health “Big Data” is extremely privacy sensitive, using it responsibly is key to establish trust and unlock the potential of this data for the health challenges facing Dutch society now and in the future. One of the unique characteristics of Big Data in health is that it is extremely partitioned across different entities. Citizens, hospitals, insurers, municipalities, schools, etc. all have a partition of the data and nobody has the complete set. Sharing across these entities is not easy due to administrative, political, legal-ethical and technical challenges.
In this project, we will establish a scalable technical and governance framework which can combine access- restricted data from multiple entities in a privacy-preserving manner. From Maastricht UMC+, we will use clinical, imaging and genotyping data from the Maastricht study, an extensive (10.000 citizens) phenotyping study that focuses on the aetiology of type 2 diabetes. From Statistics Netherlands (CBS), which hosts some of the biggest and most sensitive datasets of the Netherlands, we will use data pertaining to morbidity, health care utilization, and mortality. Our driving scientific use case is to understand the relation between diabetes, lifestyle, socioeconomic factors and health care utilization, which will inform guidelines with major public health impact.
The work plan is divided into two interlocking work packages. A Technical WP will first involve the development of a technical framework to make the Maastricht Study and CBS data FAIR – Findable, Accessible, Interoperable, and Reusable. We will couple FAIR data to a federated learning framework based on the “Personal Health Train” approach to learn target associations from the data in a privacy-preserving manner. The second WP will focus on Ethics, Law and Society Issues (ELSI), in which we will first set up a governance framework including the legal and ethics basis for the processing of the data held by the chosen test sites is sufficient for this specific scientific case and then a broader and scalable governance structure is developed to define and underpin the responsible use of Big Data in health.
Project Team
- IDS: Michel Dumontier, Claudia van Oppen, Chang Sun, Lianne Ippel, Alexander Malic, Seun Adekunle
- ELSI: David Townend, Birgit Wouters
- Maastro: Andre Dekker, Johan van Soest
- CBS: Bob van der Berg, Marco Puts, Susan van Dijk, Olo Mussmann
- De Maastricht Study: Annemarie Koster, Carla van der Kallen
Poster
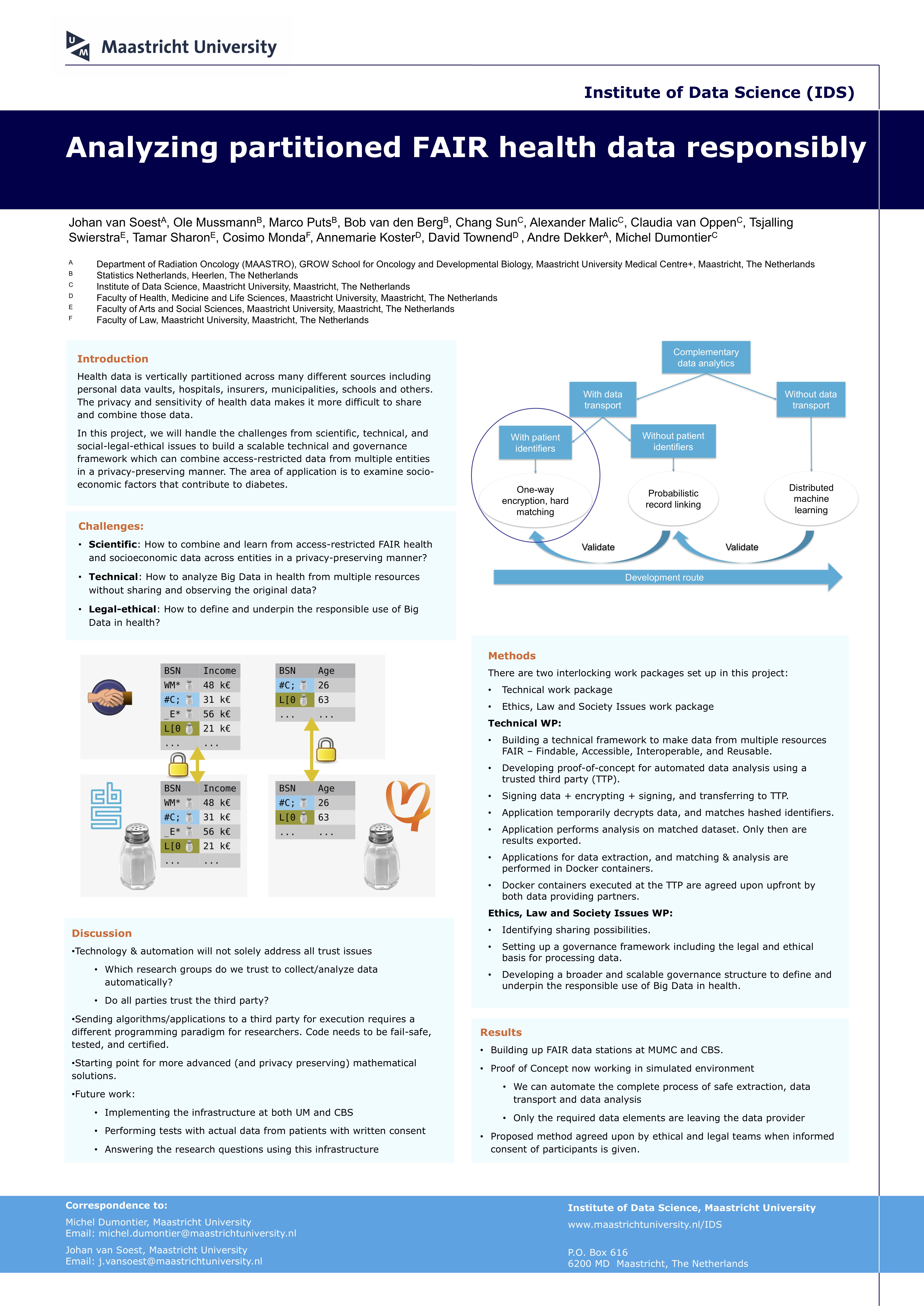