Meet Prof. Nava Tintarev, full professor of Explainable Artificial Intelligence
Prof. Nava Tintarev joined Maastricht University in October of last year. She focuses on Explainable Artificial Intelligence, which has an important societal component to it: “My take on things is much more user-centered than what you see in a lot of computer science departments.”
You may not know what a recommender system is, but you have almost certainly encountered one. “It’s the type of automated systems used by companies like Amazon and Spotify, which look at your previous profile and try to suggest something in the future”, says Prof. Tintarev.
As a recently appointed full professor in Explainable Artificial Intelligence, Nava Tintarev is a leading expert in human-computer interaction. Her work focuses on artificially intelligent systems that give advice. ‘Advice’ is to be taken broadly here: beyond recommending what to buy, see or hear next, it can for instance also refer to how search engines prioritize search results for specific users.
Explaining the unexplained
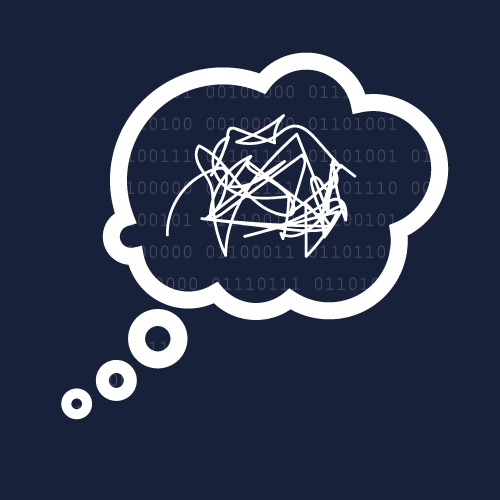
Most artificially intelligent systems are notorious for their black box-like nature: they tend to make predictions based on previous data, but won’t reveal how they arrived at their conclusions. Where a person might say ‘I recommend you look into cat supplies, because you searched the internet for pet shelters and cat gifs all of last week’, an algorithm’s reasoning can be much more sophisticated – and entirely opaque.
That lack of transparency comes at a price.
What if an algorithm is biased, but used for important things like your news consumption – thereby informing your opinions about disputed topics like face masks and vaccinations? And what if a computer’s biases only amplify yours, but you don’t know that it’s happening?
The field of Explainable Artificial Intelligence devises methods to generate insights into why AI recommends or takes particular actions. Explanations may for instance be presented in natural language or through using intelligent interactive interfaces. Such explanations are widely considered a key requirement for the responsible use of artificial intelligence in society.
Recommender systems
Back to recommender systems. Since they pop up in all sorts of settings, working on them takes on varied contexts as well. “I do a lot of work on recommender systems,” says Prof. Tintarev, “for instance in the music domain. Maybe things like the weather, your mood, and activity level change the kind of music you enjoy. The amount of interactions and explanations that you could need in these settings may also differ. You can adapt the recommender system and the explanations they generate to these kinds of things.”
“Right now, my team is working on explaining automated group recommendations. These will often require a compromise. If someone is not getting their first choice, how can we use explanations to keep the group happy and smooth those rough edges?”
Opening Pandora’s box
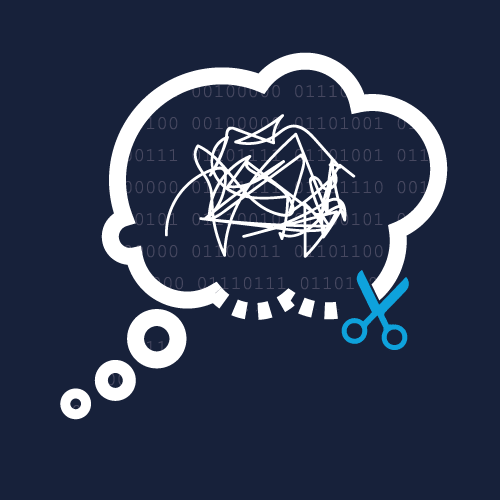
But while Prof. Tintarev initially mentions tourist and music recommendations, smoothing edges through explanations can also tackle important societal goals. Recommender systems have been under scrutiny for the risk of promoting so-called ‘filter bubbles’: online spaces where users are served content that aligns with their viewpoints, whether through Youtube, Facebook, or a different platform.
Prof. Tintarev: “I’m concerned about the fact that we are becoming more polarized. That we aren’t exposed to viewpoints that differ from our own – even when we want to be."
"The goal is not necessarily to agree with those other viewpoints, but to be aware that they exist. How do we create interaction in a way that helps people make informed decisions? Many of my current projects, also in collaboration with industry, examine online information and diversity of viewpoints. In a project co-funded by IBM, we are for example looking into search results. Does it matter which results are in the list? Or how they are ordered and displayed? How does this influence user behavior and attitudes?” The end goal, she stresses, is a broad awareness of their information consumption.
Not your average computer scientist
“My vision of Explainable Artificial Intelligence is one that is human understandable”, prof. Tintarev concludes. “We don’t only need to be able to automatically generate explanations, we also need to see when they help people make better informed decisions. That requires empirical work, like evaluations with users. It also requires understanding the circumstances: when are explanations needed and useful? What do we need to change and how should we adapt the explanations?”
“My take on things is much more user-centered than what you see in a lot of computer science departments. It’s half psychology, half computer science.” Needless to say, we’re excited to have Prof. Tintarev on our team.
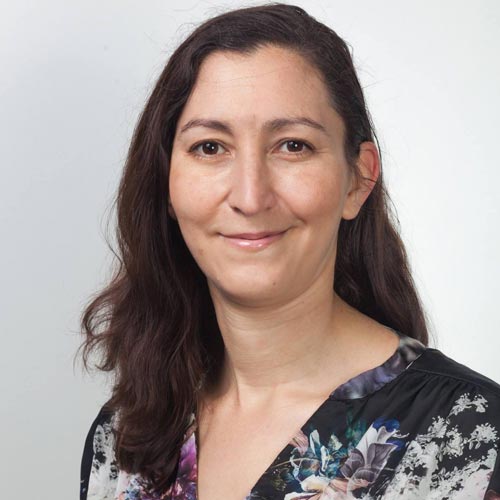
Department of Data Science and Knowledge Engineering
Biography
Prof. Dr. Nava Tintarev leads and contributes to several national and international projects in the field of human-computer interaction in artificial advice-giving systems, specifically developing the state-of-the-art for automatically generated explanations and explanation interfaces.
These include projects funded by IBM, Twitter, and the European Commission. She has collaborated with private and public organizations including Inspectie Leefomgeving en Transport (ILT), CGI/Prorail, Capgemini/Unilever, Porsche, Blendle, PersGroep, and FDMedia. In addition, Prof. Tintarev regularly shapes international research programmes and organizes workshops relating to responsible data science. ACM, the Association for Computing Machinery, recognized her as senior member in 2020.
Prof. Tintarev was appointed Full Professor and Chair of Explainable Artificial Intelligence at UM’s Faculty of Science and Engineering on October 1, 2020. She is embedded in FSE’s Department of Data Science and Knowledge Engineering. She is also a Visiting Professor in the Software Technology department at TU Delft.
Also read
-
"I am proud that our new Circular Plastics group published its first completely in-house research," Kim Ragaert says. She founded the research group three years ago, when she moved to Maastricht. Her work has laid the foundations for many innovations in the field of plastic recycling, and she is...
-
Gerco Onderwater investigates the flavour of the universe while guarding the flavour of the Maastricht Science Programme. On 31 May, during his inaugural lecture, he provided a pre-taste of his work in Maastricht.
-
Lee Bouwman, a vascular surgeon and endowed professor of Clinical Engineering, specialises in the implementation of groundbreaking healthcare technologies. The key to success, he says, lies in the collaboration between engineers and clinicians. This approach has already resulted in a range of...