Affective & Visual Computing Lab (AVCL)
How can a machine interpret human behavior as accurately as possible? And how can human behavior be used to personalize the way a machine works? Research within AVCL aims to enable the automated sensing of behaviors, emotions, and intents to improve people’s daily lives. AVCL makes use of the latest advances in Computer Vision, Natural Language Processing and Artificial Intelligence.
This is an old research theme of the Department of Data Science and Knowledge Engineering (DKE). DKE has become the Department of Advanced Computing Sciences.
The automated sensing of human behavior, activities and emotions can significantly improve quality of life. This is the case in various domains. For example, being able to analyze people’s emotions and personalities – by recognizing their facial expressions, their posture or motion analysis – can help in domains like education and marketing. Similarly, modelling people’s activities in smart environments (for example through cameras, microphones and other sensors) can revolutionize fields such as integrated healthcare and domestic energy management.
Such applications require machines to interpret human behavior correctly, and to do so in appropriate situational context (‘in the wild’). To achieve this, state-of-the-art methods need to analyze and mesh together data from sensors such as video cameras and microphones, wearable devices, and other sources including text and application-dependent (context) information.
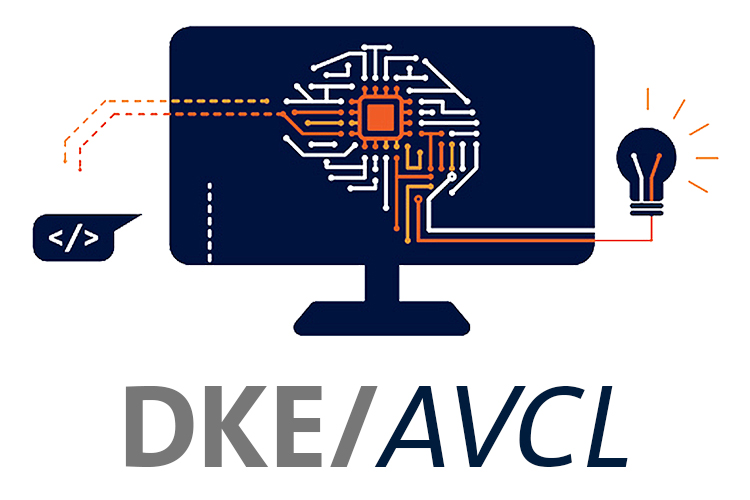
Scope
- Affective Computing
- Computer Vision
- Natural Language Processing
- Deep Learning
- Machine Translation
- Social Machine Learning
The AVCL website is under construction: AVCL was previously embedded in the Robots, Agents and Interaction (RAI) group.
Go to RAI website
Research focus and application
The Affective & Visual Computing Lab builds techniques that allow machines to combine data from different sources and interpret human behavior as accurately as possible. The scope of the lab encompasses both fundamental research and research into a wide range of innovative applications.
AVCL researchers are currently working in the domains of multimodal emotion and personality recognition in the wild (e.g. educational settings and beyond), activity recognition for senior citizens (using computer vision, health records, digital interactions, ambient sensors), knowledge transfer in affective computing, (visual) event recognition and text retrieval.
AVCL projects are tested in real operational environments including schools, hospitals, daily care centers and home environments.
Affective & Visual Computing in practice
Dr. Stelios Asteriadis discusses how computers can take emotions into account when teaching
Researchers
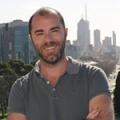
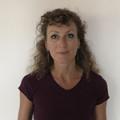
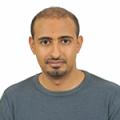
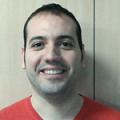
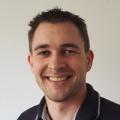
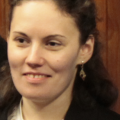
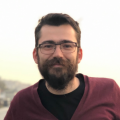
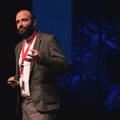
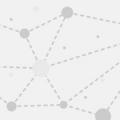
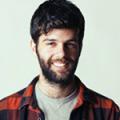
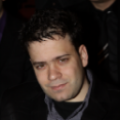
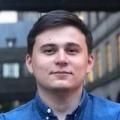
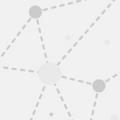
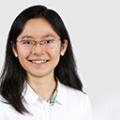
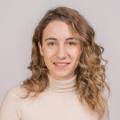
Highlighted publications
- Alvanitopoulos, P., Diplaris, S., de Gelder, B., Shvets, A., Benayoun, M., Koulali, P., Moghnieh, A., Shekhawat, Y., Stentoumis, C., Hosmer, T., Anadol, R., Borreguero, M., Martin, A., Sciama, P., Avgerinakis, K., Petrantonakis, P., Briassouli, A., Mille, S., Tellios, A., ... Kompatsiaris, I. (2019). MindSpaces: Art-driven Adaptive Outdoors and Indoors Design. In 9th International Conference on Digital Presentation and Preservation of Cultural and Scientific Heritage (DiPP): DiPP2019 (Vol. 9, pp. 391-400). Digital presentation and preservation of cultural and scientific heritage
- Alvarez, F., Popa, M., Solachidis, V., Hernandez-Penaloza, G., Belmonte-Hernandez, A., Asteriadis, S., Vretos, N., Quintana, M., Theodoridis, T., Dotti, D., & Daras, P. (2018). Behavior Analysis through Multimodal Sensing for Care of Parkinson's and Alzheimer's Patients. Ieee Multimedia, 25(1), 14-25. https://doi.org/10.1109/MMUL.2018.011921232
- Athanasiadis, C., Amestoy, M., Hortal, E., & Asteriadis, S. (2020). e3Learning: A Dataset for Affect-Driven Adaptation of Computer-Based Learning. Ieee Multimedia, 27(1), 49-60. https://doi.org/10.1109/mmul.2019.294571
- Athanasiadis, C., Hortal, E., & Asteriadis, S. (2020). Audio-Based Emotion Recognition Enhancement Through Progressive GANS. In 2020 IEEE International Conference on Image Processing (ICIP) (pp. 236-240). IEEE. https://ieeexplore.ieee.org/document/9190959
- Athanasiadis, C., Hortal, E., & Asteriadis, S. (2020). Audio–visual domain adaptation using conditional semi-supervised Generative Adversarial Networks. Neurocomputing, 397, 331-344. https://doi.org/10.1016/j.neucom.2019.09.106
- Bauer, T., Devrim, E., Glazunov, M., Jaramillo, W. L., Mohan, B., & Spanakis, G. (2020). #MeTooMaastricht: Building a chatbot to assist survivors of sexual harassment. In P. Cellier, & K. Driessens (Eds.), Machine Learning and Knowledge Discovery in Databases: ECML PKDD 2019. Communications in Computer and Information Science (Vol. 1167, pp. 503-521). Springer International Publishing. Communications in Computer and Information Science Vol. 1167 https://doi.org/10.1007/978-3-030-43823-4_41
- Dotti, D., Ghaleb, E., & Asteriadis, S. (2020). Temporal Triplet Mining for Personality Recognition. In Struc, & F. GomezFernandez (Eds.), 2020 15th IEEE International Conference on Automatic Face and Gesture Recognition (FG 2020) (Vol. 1, pp. 379-386) https://doi.org/10.1109/fg47880.2020.00024
- Dotti, D., Popa, M., & Asteriadis, S. (2020). A hierarchical autoencoder learning model for path prediction and abnormality detection. Pattern Recognition Letters, 130, 216-224. https://doi.org/10.1016/j.patrec.2019.06.030
- Dotti, D., Popa, M., & Asteriadis, S. (2020). Being the center of attention: A Person-Context CNN framework for Personality Recognition. Transactions on Interactive Intelligent Systems, 10(3), [19]. https://doi.org/10.1145/3338245
- Ghaleb, E., Niehues, J., & Asteriadis, S. (2020). Multimodal Attention-Mechanism For Temporal Emotion Recognition. In 2020 IEEE International Conference on Image Processing (ICIP) (pp. 251-255) https://doi.org/10.1109/icip40778.2020.9191019
- Ghaleb, E., Popa, M., & Asteriadis, S. (2019). Multimodal and Temporal Perception of Audio-visual Cues for Emotion Recognition. In 8th International Conference on Affective Computing & Intelligent Interaction (ACII 2019), Cambridge, United Kingdom
- Khaertdinov, B., Ghaleb, E., & Asteriadis, S. (2021). Deep Triplet Networks with Attention for Sensor-based Human Activity Recognition. In 2021 IEEE International Conference on Pervasive Computing and Communications (PerCom) (pp. 1-10). [9439116] IEEE Xplore. https://doi.org/10.1109/PERCOM50583.2021.9439116
- Koneru, S., Liu, D., & Niehues, J. (2021). Unsupervised Machine Translation On Dravidian Languages. In Proceedings of the First Workshop on Speech and Language Technologies for Dravidian Languages (pp. 55-64). Association for Computational Linguistics. https://aclanthology.org/2021.dravidianlangtech-1.7.pdf
- Liu, D., Spanakis, G., & Niehues, J. (2020). Low-Latency Sequence-to-Sequence Speech Recognition and Translation by Partial Hypothesis Selection. In Proceedings of Interspeech 2020 (pp. 3620-3624) https://doi.org/10.21437/Interspeech.2020-2897
- Meyers, M., Weiss, G., & Spanakis, G. (2020). Fake News Detection on Twitter Using Propagation Structures. In M. van Duijn, M. Preuss, V. Spaiser, F. Takes, & S. Verberne (Eds.), Disinformation in Open Online Media (pp. 138-158). Springer International Publishing. https://doi.org/10.1007/978-3-030-61841-4_10
- Mino, A., & Spanakis, G. (2018). LoGAN: Generating Logos with a Generative Adversarial Neural Network Conditioned on Color. In 17th IEEE International Conference on Machine Learning and Applications, ICMLA 2018, Orlando, FL, USA, December 17-20, 2018 (pp. 965-970) https://doi.org/10.1109/ICMLA.2018.0015
- Montulet, R., & Briassouli, A. (2019). Deep Learning for Robust end-to-end Tone Mapping. In British Machine Vision Conference Proceedings
- Montulet, R., & Briassouli, A. (2020). Densely Annotated Photorealistic Virtual Dataset Generation for Abnormal Event Detection. In Proceedings of the International Conference on Pattern Recognition, ICPR 2020: ICPR FGVRID Workshop: Fine-Grained Visual Recognition and re-Identification
- Mulsa, R. A. C., & Spanakis, G. (2020). Evaluating Bias In Dutch Word Embeddings. In M. R. Costa-jussà, C. Hardmeier, W. Radford, & K. Webster (Eds.), Proceedings of the Second Workshop on Gender Bias in Natural Language Processing (pp. 56-71). Association for Computational Linguistics. https://www.aclweb.org/anthology/volumes/2020.gebnlp-1/
- Niehues J. Continuous Learning in Neural Machine Translation using Bilingual Dictionaries. In Proceedings of the 16th Conference of the European Chapter of the Association for Computational Linguistics (EACL) 2021 April 19 (pp. 830-840). Association for Computational Linguistics.
- Standen, P. J., Brown, D. J., Taheri, M., Trigo, M. J. G., Boulton, H., Burton, A., Hallewell, M. J., Lathe, J. G., Shopland, N., Gonzalez, M. A. B., Kwiatkowska, G. M., Milli, E., Cobello, S., Mazzucato, A., Traversi, M., & Hortal, E. (2020). An evaluation of an adaptive learning system based on multimodal affect recognition for learners with intellectual disabilities. British Journal of Educational Technology, 51(5), 1748-1765. https://doi.org/10.1111/bjet.13010