Making Sense of Uncertainty
Uncertainty can make our lives difficult. If you are looking to sign an energy contract now (mid-2023), you may find it difficult to choose between a fixed rate and a variable rate since we are unsure if the energy price will rise or fall. Historically, uncertainty always spikes during crises. According to the Global Economic Policy Uncertainty Index (Figure 1), the level of uncertainty during the 2000 Dot-Com crisis was around 170, rose to over 200 during the 2008 Global Financial Crisis, and peaked at 440 during the 2020 Covid Crisis[1]. This implies a growing magnitude of uncertainty, making us more vulnerable. However, what do we really know about uncertainty? This blogpost will walk through the basic concepts of uncertainty in economics, coupled with a few takeaways from our research.
All uncertainty is not the same – there is risk and ambiguity
According to the Cambridge Dictionary, uncertainty means “a situation in which something is not known, or something that is not known or certain.” If we use this definition literally, rising uncertainty means an increase in things that we do not know. However, as economists studying uncertainty, we can be more precise. Back in 1921, Frank Knight was the first economist to propose a specific definition of what we now call ambiguity; Knightian uncertainty[2] (as opposed to the better-known concept of risk) refers to a situation in which knowing past data (and its distribution) is not helpful for us to infer the likelihood of future outcomes. For example, rolling dice is considered to be a risky event where the possible outcome ranges from a number 1 to a number 6, where each outcome has a probability of 1/6. Now, what if we receive a new dice, which is unbalanced due to some manufacturing errors? It means that the probability of each outcome may not be 1/6 and we do not know the true probability - this is known as ambiguity. It is well-documented that people dislike both risk and ambiguity and, in economics, these conditions are referred to as risk aversion and ambiguity aversion respectively.
People hate ambiguity more than risk
40 years after Frank Knight purposed the concepts of risk and ambiguity, Daniel Ellsberg published a seminal paper showing that people respond to risk and ambiguity differently[3]. To illustrate Ellsberg’s argument, let us do a thought experiment. Imagine a box containing 90 balls, 30 of which are orange and the other 60 of which are either black or blue. The exact numbers of black and blue balls are unknown. If you were to pick a colour and draw a ball from the box, and the ball matches your chosen colour, then you receive 1000 Euros. Which colour would you choose?
Studies show that people are far more likely to select orange over blue or black. However, this is puzzling since there is no rational ground to do so. For instance, if we assume the number of black (blue) balls is smaller than orange balls, we would choose orange over black (blue). But the combination of black and blue already makes 60, meaning there must be more blue (black) than orange balls. This means that it is more rational to pick blue (black) instead of orange. However, this is not the most chosen option in reality – hence the famous label “Ellsberg’s paradox”. People prefer orange to blue and black simply because they know the probability of obtaining one is 1/3 whereas the probabilities for black and blue balls are unknown. All this is to say that we generally prefer risk to ambiguity.
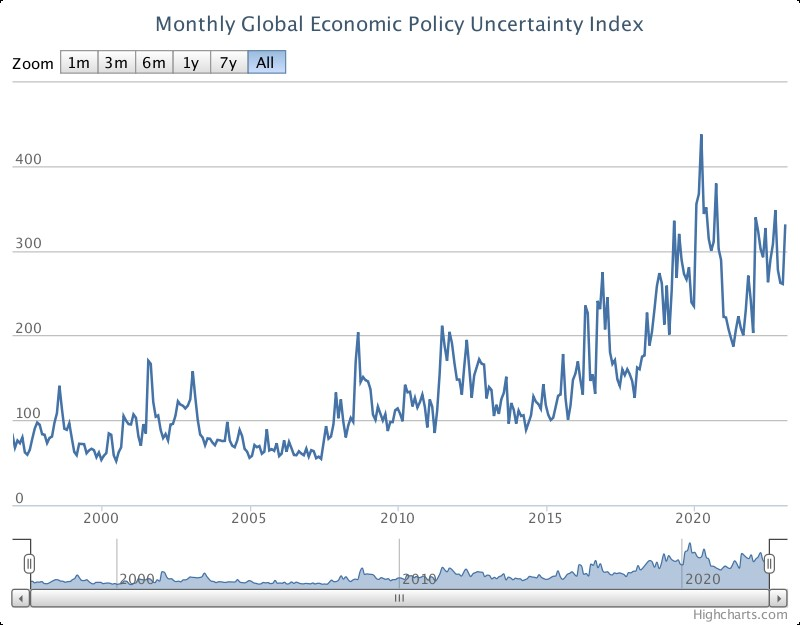
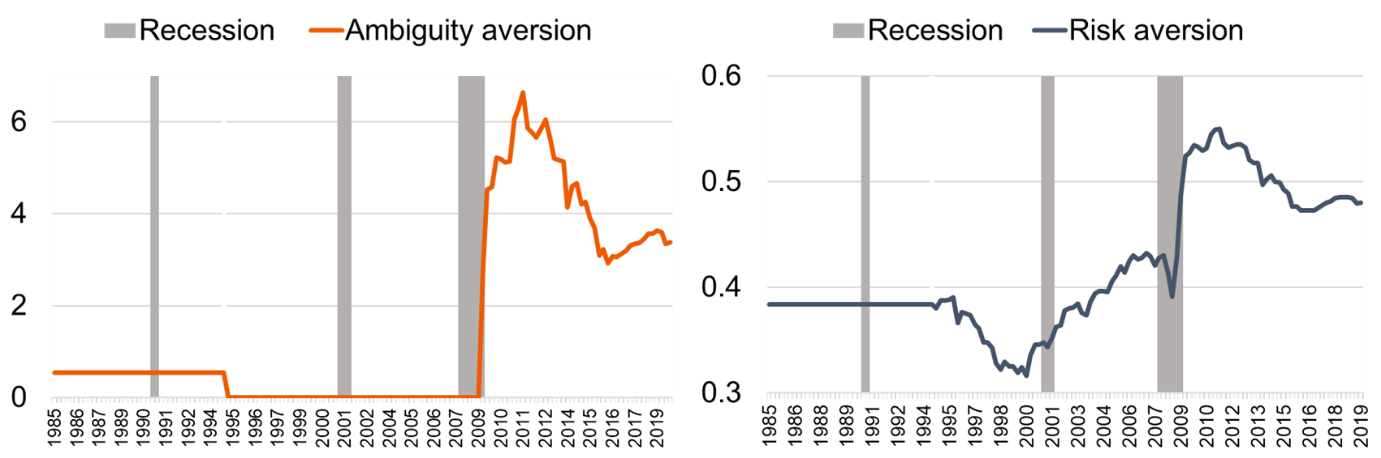
Risk often makes us feel more uncertain; however, ambiguity can make us feel either more certain or more uncertain.
For example, a dice rolling is riskier than a coin flipping because a dice can have six outcomes while a coin can have only two outcomes. Thus, the outcome of dice rolling is more uncertain than the outcome of coin flipping. However, ambiguity can have the opposite effect, potentially making people more certain. Research suggests that when making decisions under ambiguity, people need a narrative (say, a specific pattern) in their minds in order to feel certain. In an experiment by Piccillo and Van Den Hurk, participants were shown ambiguous pictures and asked if they could detect a human face in these pictures. Some pictures actually did contain a (hard to see) human face, while others did not. Surprisingly, individuals who are more ambiguity averse tend to see more faces even in pictures without one[4]. Our analysis of household surveys uncovers consistent results. When the economic policy uncertainty index is high, the households in the US and in the Netherlands predict a significantly lower income and claim to have a significantly lower subjective uncertainty, by showing more confidence in their forecast[5]. In other words, when uncertainty increases, people are more pessimistic, anticipating decreased incomes and becoming more precise in their forecast, and more assured of their forecast.
The levels of risk aversion and ambiguity aversion are evolving over time, and they rose significantly in the last fifteen years.
We have estimated risk and ambiguity aversion in the US using a macroeconomic model and found that these changes in attitudes are closely associated with economic crises[6]. Figure 2 shows our results. In the late 1990s, financial speculation in the new IT businesses was out of control, with the NASDAQ index surging from 2k to 5k in one year from 1999 to early 2000. People were relatively less risk-averse during this time, and the perception of risk was low. However after they realized that many of these IT companies were overvalued, the bubble burst, triggering an economic recession, and risk aversion increased significantly. Notably, throughout these fluctuations ambiguity aversion remained constant.
However the Global financial crisis (GFC) in 2008 was different. By the end of the GFC, both risk and ambiguity aversion had a sharp increase, and they have yet to return to pre-GFC levels. An increase in ambiguity aversion, as discussed previously, implies that people became more pessimistic and possibly more certain in their pessimism after the GFC. This could be one of the reasons why the economic recovery from GFC was slower than the recovery from the Dot-com crisis when ambiguity aversion was lower. It would be interesting to see a study of this kind estimating risk and ambiguity aversion during the COVID-19 pandemic, which is the highest level of uncertainty the world has faced since World War II.
“Uncertainty is an uncomfortable position. But certainty is an absurd one.” - Voltaire
As economists, we study how uncertainty affects the economy, and so it is important to understand how people, the main players in the economy, respond to uncertainty. Distinguishing between risk and ambiguity has helped us gain deeper insights into the relationship between individuals and uncertainty. We now know that:
- People generally dislike ambiguity more than risk.
- Risk can make us feel more uncertain, whereas ambiguity can either make us feel more certain or more uncertain.
- The levels of risk aversion and ambiguity aversion are changing over time and are often related to economic crises.
Voltaire's quote emphasizes the purpose of this blog: we may not be able to avoid uncertainty, but we can do our best to make sense of it.
[1] Baker, S. R., N. Bloom, and S. J. Davis. (2016). “Measuring Economic Policy Uncertainty”, Quarterly Journal of Economics, 131, 1593-1636.
[2] Knight, F. H. (1921). “Risk, Uncertainty and Profit”, SSRN Scholarly Paper ID 1496192, Social Science Research Network, Rochester, NY.
[3] Ellsberg, D. (1961). “Risk, Ambiguity, and the Savage Axioms”, Quarterly Journal of Economics. 75 (4), 643–669.
[4] Piccillo, G. and J. Van Den Hurk. (2020). “The Surprising Effect of Social Distancing on Our Perception: Coping with Uncertainty”, CEPR Press (20), 132.
[5] Piccillo, G. and P. Poonpakdee. (2021). “Effects of Macro Uncertainty on Mean Expectation and Subjective Uncertainty: Evidence from Households and Professional Forecasters”, CESifo Working Paper Series
[6] Piccillo, G. and P. Poonpakdee. (2023). “Ambiguous Business Cycles, Recessions and Uncertainty: quantitative analysis”
Also read
-
Global recognition for SBE’s Executive Education by Financial Times
We are proud to announce that Maastricht University School of Business and Economics (SBE) has secured an impressive global ranking of 84th in the Financial Times Open-Enrolment Executive Education Ranking 2025.
-
SBE Researchers Awarded in NWO SGW Open Competition XS
The School of Business and Economics (SBE) at Maastricht University proudly announces that all three research proposals submitted to the latest NWO SGW Open Competition XS have been awarded funding. This exceptional outcome highlights the high calibre of SBE research and its relevance to societal...
-
Designing a Resilient Plastics Economy: Take-Aways from the MORSE Panel Debate
On 27 January 2025, MORSE’s Research Day convened a high-level panel—featuring Arjen Wittekoek, Nelleke van der Puil and Laura Nieboer—to unpack the Dutch plastics-recycling crisis (in 2024 six recyclers went bankrupt in 2024). The discussion highlighted how Extended Producer Responsibility...