The bigtime software toolbox: an Elinor Ostrom grant project
This article was written by Dr. Ines Wilms, an assistant professor in Econometrics, and Enrico Wegner, an Economic and Financial Research (EFR) master student, at Maastricht University’s School of Business and Economics. In this piece, Ines and Enrico introduce the bigtime software toolbox they have developed through the Elinor Ostrom Fund.
Statistical Learning from Big Data
Big Time Series Data are nowadays omnipresent in economics: technological advances allow, for instance, financial analysts to process intraday data on hundreds of stocks, retailers to keep records of all products purchased on each shopping trip, or macro-economists to forecast the state of the economy in real time from a large collection of time series recorded at various frequencies. While the Big Data concept has been around for years, most researchers and organizations now understand that they need an appropriate statistical toolkit to extract information from Big Data. We develop novel statistical methods that learn patterns from Big Time Series Data to allow for more confident economic making.
A software toolbox: bigtime!
"In this Elinor Ostrom grant project, I collaborate with Enrico Wegner to boost the visibility of my research among a wide and general audience of (applied) researchers, students, and business analysts by equipping them with a software toolbox. The software toolbox offers state-of-the-art methods for jointly modeling a large collection of time series and it accounts for the needs of both expert and novice users. For expert users, we provide a comprehensive open-source R-package called bigtime where my recent work (Wilms et al. 2020, 2021) is implemented. For novice users, we provide web-based applications where we guide them through basic time series concepts on which the methods rest as well as the package’s functionality. Users can discover the practical usefulness of the methods via use cases without having to program anything themselves. Feel free to have a look yourself to discover (part of) the rich world on Big Time Series Analysis here!" - Ines Wilms
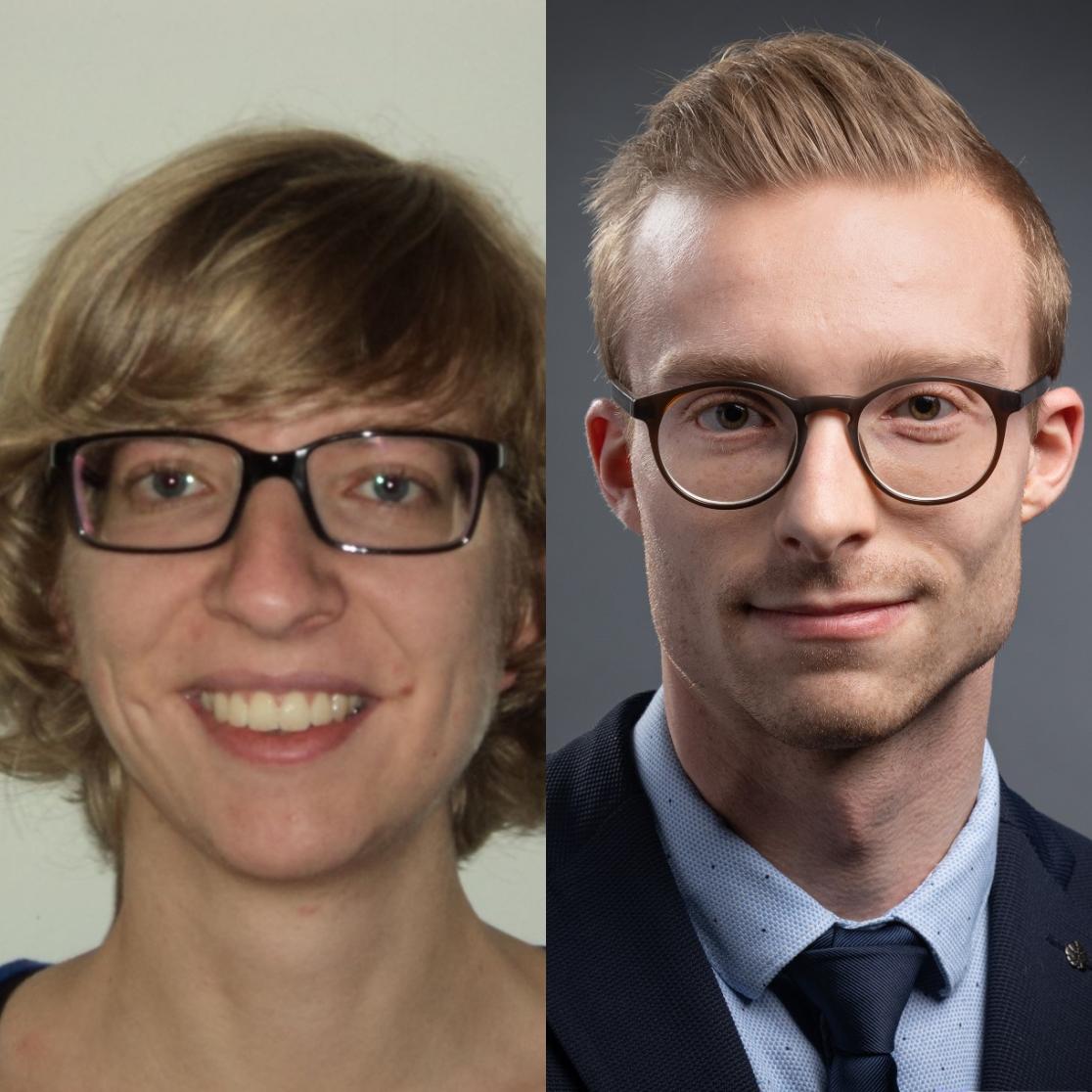
A student’s perspective
"Working with Ines on improving the R-package bigtime and developing web-based applications has turned out to be the perfect finish to this academic year. It allowed me to bring together courses such as Time Series Econometrics and High-Dimensional Econometrics, which are both taught in the first year of the Econometrics master. Although both already emphasize the importance of Big Time Series Analysis, this project extended my knowledge from these courses by introducing sparse Vector AutoRegressive (VAR) models and extensions thereof.
VAR models are usually introduced as the backbone of macro-economic modelling but are often only used with few time series. This is mostly due to VAR models becoming very high-dimensional very quickly and due to traditional methods not being able to handle such high-dimensional cases very well. Working with Ines on this project has taught me that using more advanced techniques and tools, such as bigtime can greatly improve the performance in high-dimensional VARs and can therefore allow us to model more time series at the same time. This in turn often results in new insights and better forecasts. These advantages of high-dimensional methods extend beyond only macro-economic modelling. For example, one of the web-based applications that resulted out of this project uses bigtime to model the connectedness of volatility in stock markets. Traditional methods would have not allowed us to model all markets at the same time. Using bigtime, on the other hand, allowed us to do exactly that, which resulted in a network graph showing how volatility propagates through stock markets. This is an insight that in this form could have never been obtained using traditional methods." - Enrico Wegner
Publications
Nicholson, W.B., Wilms, I., Bien, J., & Matteson, D.S. (2020). High dimensional forecasting via interpretable vector autoregression. Journal of Machine Learning Research, 21(166), 1-52.
Wilms, I., Basu, S., Bien, J., & Matteson, D.S. (2021). Sparse Identification and Estimation of Large-Scale Vector AutoRegressive Moving Averages. Journal of the American Statistical Association, doi: 10.1080/01621459.2021.1942013.
The Elinor Ostrom Fund
The Elinor Ostrom Fund, which was founded by Professor Lisa Brüggen in 2014, supports female scholars in an effort to increase diversity at Maastricht University's School of Business and Economics. |
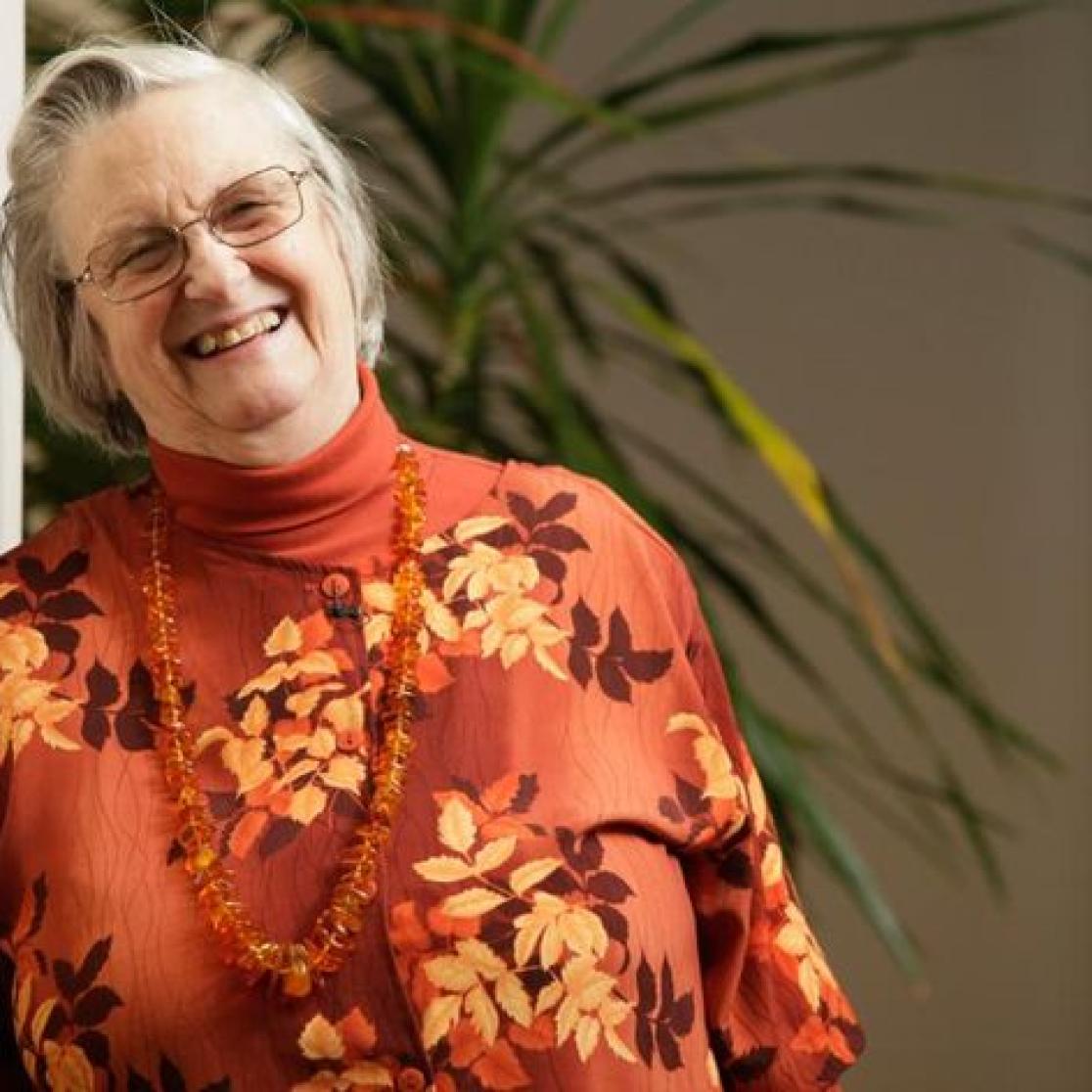
Also read
-
From Economics to Branding and Innovation: The journey of Patrick van Thiel
Patrick van Thiel’s academic journey began in Rotterdam before he found his true calling at Maastricht University in 1989. Drawn by the Problem-Based Learning (PBL) system, he quickly excelled academically, earning 90 credits in just one year. However, it wasn’t until he discovered his passion for...
-
Rethinking Higher Education in an AGI World: Reflections from the MINDS Workshop
With artificial intelligence (AI) developing at a rapid pace, conversations around its future impact are becoming increasingly urgent. While artificial general intelligence (AGI) — systems that could rival or exceed human-level performance across tasks — remains a highly debated concept, it cannot...
-
Discrimination makes women want to work less
Recent research by scientists at Maastricht University in the Netherlands and Aarhus University in Denmark shines a new light on the gender pay gap. Discrimination makes women want to work fewer hours.