UM Data Science Research Seminar
The UM Data Science Research Seminar Series are monthly sessions organised by the Institute of Data Science, on behalf of the UM Data Science Community, in collaboration with different departments across UM with the aim to bring together data scientists from Maastricht University to discuss breakthroughs and research topics related to Data Science.
This session is organised in collaboration with the Department of Bioinformatics – BiGCaT on 14th November, 2019 from 11:45 - 1:15pm.
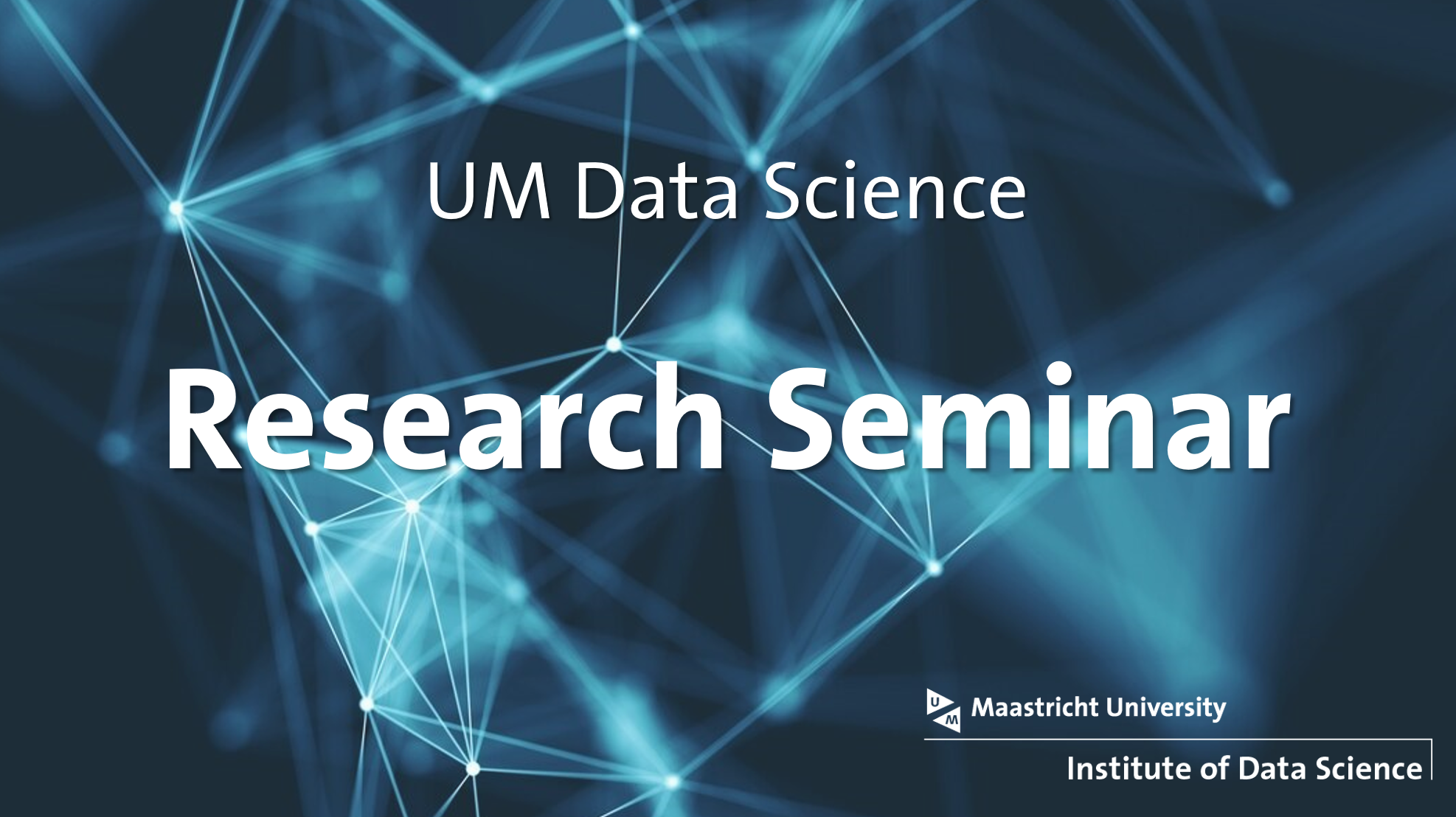
UNS 60, Co Greepzaal
Event is free and open to everyone. Lunch will be provided. Please register by 11th November, 2019.
Schedule
12.00 - 12.30
Talk by Denise Slenter
Title: Applying directed networks from biological pathways to visualise and interpret metabolomics data
Abstract: Pathway diagrams are useful for computational analysis and interpretation of large-scale experimental data, when properly modelled. Online pathway databases (e.g. WikiPathways, Reactome, LipidMaps) provide rich, intuitive models of pathways. Pathway analysis is widely adopted in the analysis of transcriptomics data. In experimental metabolomics data, however, many measured metabolites cannot be linked to the metabolites present in pathways databases. The resulting sparseness complicates the use of metabolomics data in pathway analysis. Here, we present our approach to calculate the shortest, directed paths between metabolites of interest. Using the WikiPathways RDF, we created a directed network of all metabolic reactions from WikiPathways, Reactome and LipidMaps. This network is stored in the graph database Neo4j and enriched with knowledge from ChEBI, HMDB and Wikidata. We tested our approach on several metabolomics datasets, including measurements from mass-spectrometry and NMR and several biological fluids (e.g. blood, urine), related to metabolic changes due to aging. With the current approach however, the weight of the edges within the network is not taken into account, which could be calculated with protein kinetic data. This would account for the flux through the paths, and could give a biologically more plausible subnetwork. We are exploring linking our metabolic pathways to flux and kinetics data, integrated with machine learning approaches to capture the dynamics of biological directed networks, and are interested in machine learning approaches to detect new pathways from experimental data.
12.30 - 1:00
Talk by Dr. Egon Willighagen
Title: Wikidata and Scholia as a hub linking chemical knowledge
Abstract: Making chemical databases more FAIR (findable, accessible, interoperable, and reusable) benefits computational chemistry and cheminformatics. We here discuss Wikidata, a sister project of Wikipedia but with one big difference: it is a machine readable database, making it far more useful for interoperability of molecular databases in systems biology. Thanks to the ikidata:WikiProject Chemistry community, there is a growing amount of information about chemical compounds: Wikidata currently has over 160 thousand chemical compounds, of which more than 96% is associated with InChIKeys and has more than 73 thousand CAS registry numbers. Ongoing work by this WikiProject includes capturing chemical classes and chemical compounds in the various Wikipedias as machine readable data. Other projects include covering human drugs, MeSH Chemicals and Drugs, and volatile organic compounds. This work is supported by the many tools around Wikidata. We here introduce our contributions to the WikiProject Chemistry to support FAIR-ification of open chemical knowledge. For example, we proposed new Wikidata properties to annotate compounds with external database identifiers for the EPA CompTox Dashboard, the SPLASH, MassBank, and LIPID MAPS. Furthermore, we used a combination of Bioclipse (via the Bacting project) and QuickStatements to add missing chemical compounds for biological pathways from WikiPathways. Finally, we introduce an extension of Scholia, visualizing data about compounds and compound classes, including external identifiers, physicochemical properties, and an overview of the literature from which the knowledge is derived.
For questions or concerns, please contact us via info-ids@maastrichtuniversity.nl.
Organizers
Department of Bioinformatics Team
- Denise Slenter
- Egon Willighagen
- Martina Summer-Kutmon