Research
MaCSBio strives to perform cutting edge research in the interdisciplinary field of Systems Biology to create a “Digital Twin”, a set of computational and mathematical models based on biological evidence that will help to understand and predict human systems. Research projects at MaCSBio focus on modelling across multiple scales of time and space, tackling areas that are highly relevant for society:
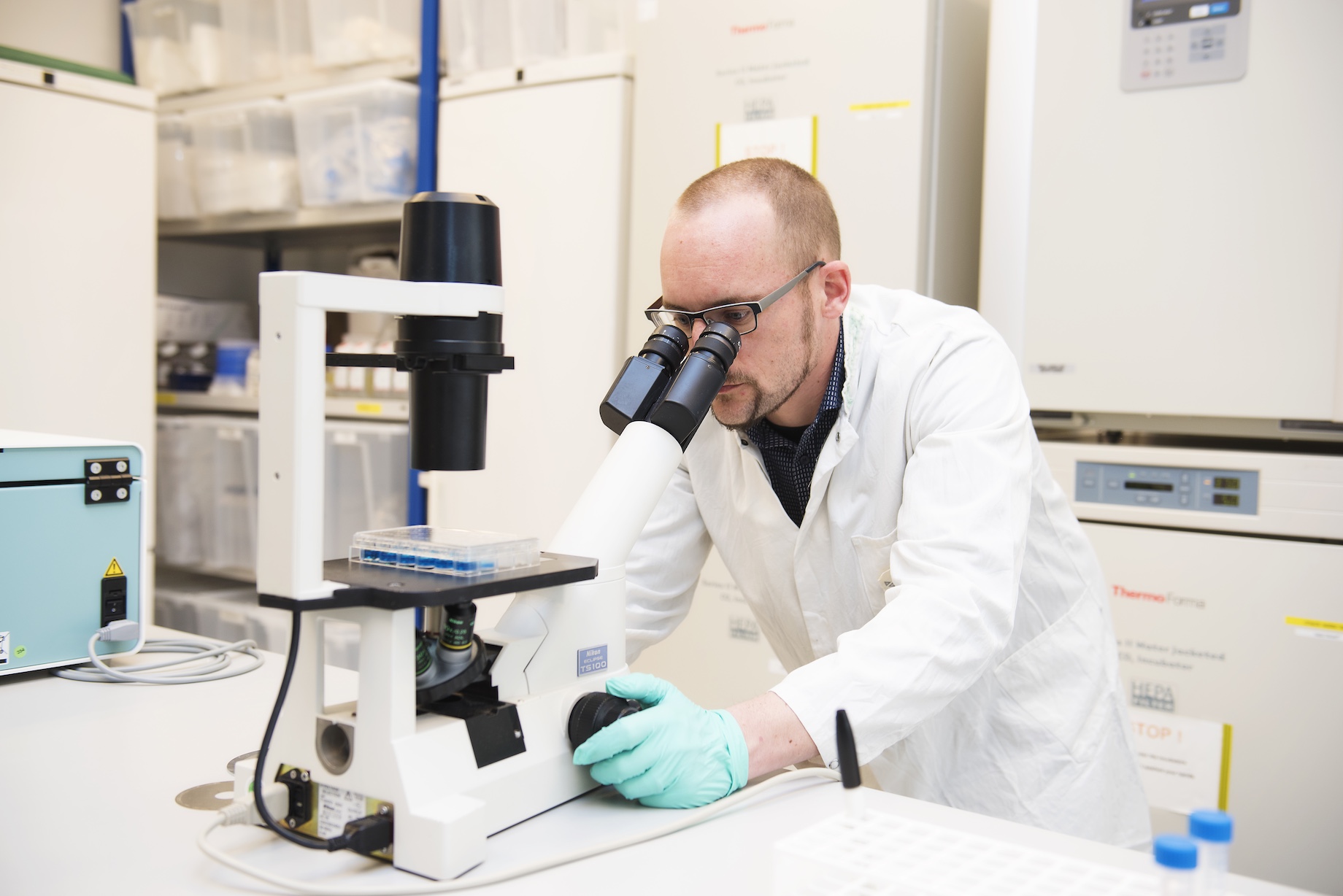