Quantum Machine Learning enters the fray in CERN’s LHCb experiment
In a recent article in the Journal of High Energy Physics (JHEP), the LHCb collaboration reports the application of Quantum Machine Learning for identifying properties of so-called jets: streams of particles that result from particle collisions.
It is the first paper to describe the application of quantum computing to the identification of jets originating from beauty quarks or anti-quarks, a type of particle of particular interest to the LHCb experiment.
Underground particle accelerator
The LHCb experiment is one of the experiments at CERN’s Large Hadron Collider. This massive underground research facility replicates conditions shortly after the Big Bang by smashing particles into each other at nearly the speed of light. The resulting collisions hold all sorts of keys to further our understanding of matter and the universe in general.
With dizzying numbers of collisions occurring every second, physicists rely on state-of-the-art artificial intelligence to identify the specific particles that interest them. Machine learning has earned its place as a key method in this line of work.
From conventional machine learning to quantum machine learning
The authors now investigate the use of Quantum Machine Learning – a new iteration of the field, which employs principles from quantum computing – to tag jets containing particles that are of particular interest to the LHCb experiment. Using simulated datasets, the researchers compare the performance of more classical approaches to that of quantum algorithms. In some scenarios, these performed comparable to their conventional counterparts. As such, this is a promising first exploration of what quantum algorithms could do for big physics experiments.
Publication
This work represents the first application of quantum computing to the identification of jets that originate from beauty quarks or anti-quarks.
The work was performed at the University of Padua in Italy by the LHCb Data Processing & Analysis Group, in which Maastricht University takes part. Co-corresponding author Davide Nicotra has since joined Maastricht University as a PhD student, where he will continue to work on quantum computing applications for LHCb particle tracking challenges.
Article (open access): Alessio Gianelle, Patrick Koppenburg, Donatella Lucchesi, Davide Nicotra, Eduardo Rodrigues, Lorenzo Sestini, Jacco de Vries and Davide Zuliani. Quantum Machine Learning for b-jet charge identification. J. High Energ. Phys. 2022, 14 (2022). https://doi.org/10.1007/JHEP08(2022)014
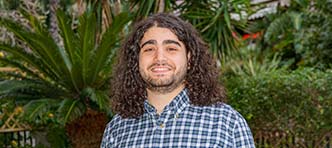
Co-corresponding author Davide Nicotra: "When I started working on my master’s thesis project about quantum computing for jet identification at the LHCb experiment, I was so excited to explore this new field of research. This work represents a first, preliminary step towards broader usage of quantum computing as a powerful tool for research - and also a personal achievement. I feel honoured to continue my research in this field as a PhD student at Maastricht University, in which I have found a very stimulating environment to pursue my studies."